The researchers hope that the model, based on a huge dataset from Denmark and the technology that powers large language models like ChatGPT, can start a public debate about the power of these tools and how they should and shouldn't be used. .
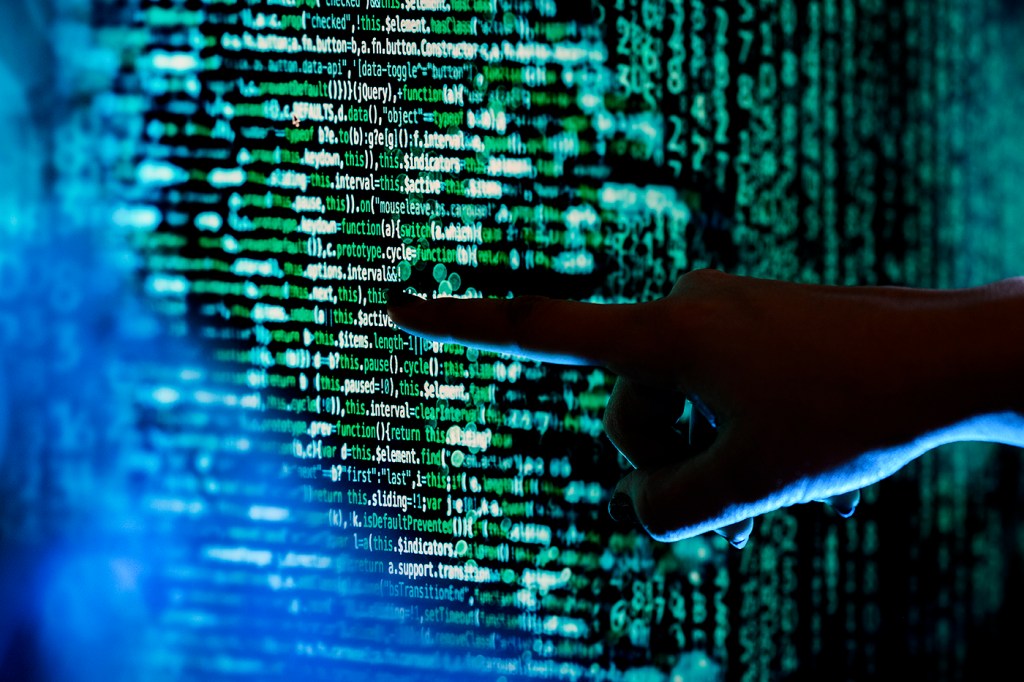
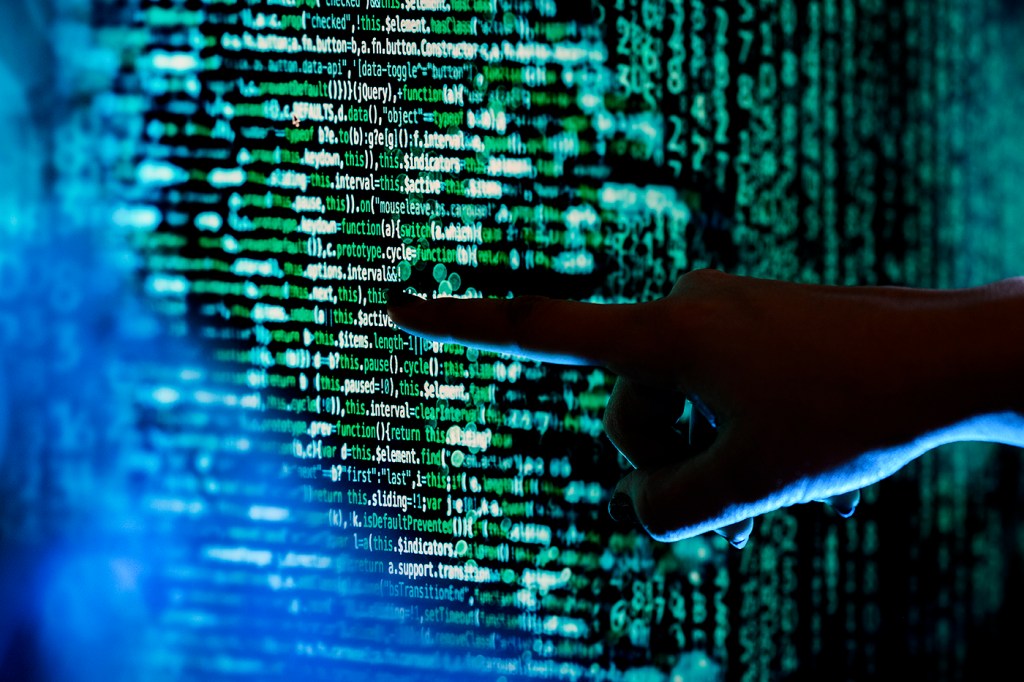
A Northeastern researcher and former postdoctoral fellow have created an artificial intelligence tool that uses sequences of life events — such as health history, education, work and income — to predict everything from a person's personality to their mortality.
Built using transformer models, which feed large language models (LLMs) such as ChatGPT, the new tool, life2vec, is trained on a dataset drawn from the entire population of Denmark — 6 million people. The data set was made available to the researchers only by the Danish government.
The tool the researchers built based on this complex data set is capable of predicting the future, including people's lifespans, with an accuracy that exceeds state-of-the-art models. But despite its predictive power, the team behind the research say it's best used as a basis for future work, not as an end in itself.
“Although we use prediction to evaluate how well these models are, the tool should not be used to predict real people,” he says. Tina Iliasi-Rand, professor of computer science and the inaugural President Joseph E. Aoun Professor at Northeastern University. “It's a predictive model based on a specific data set of a specific population.”
Eliassi-Rad brought her expertise in artificial intelligence to the project. “These tools allow you to look at your society in a different way: the policies you have, the rules and regulations you have,” he says. “You can think of it as a scan of what's happening on the ground.”
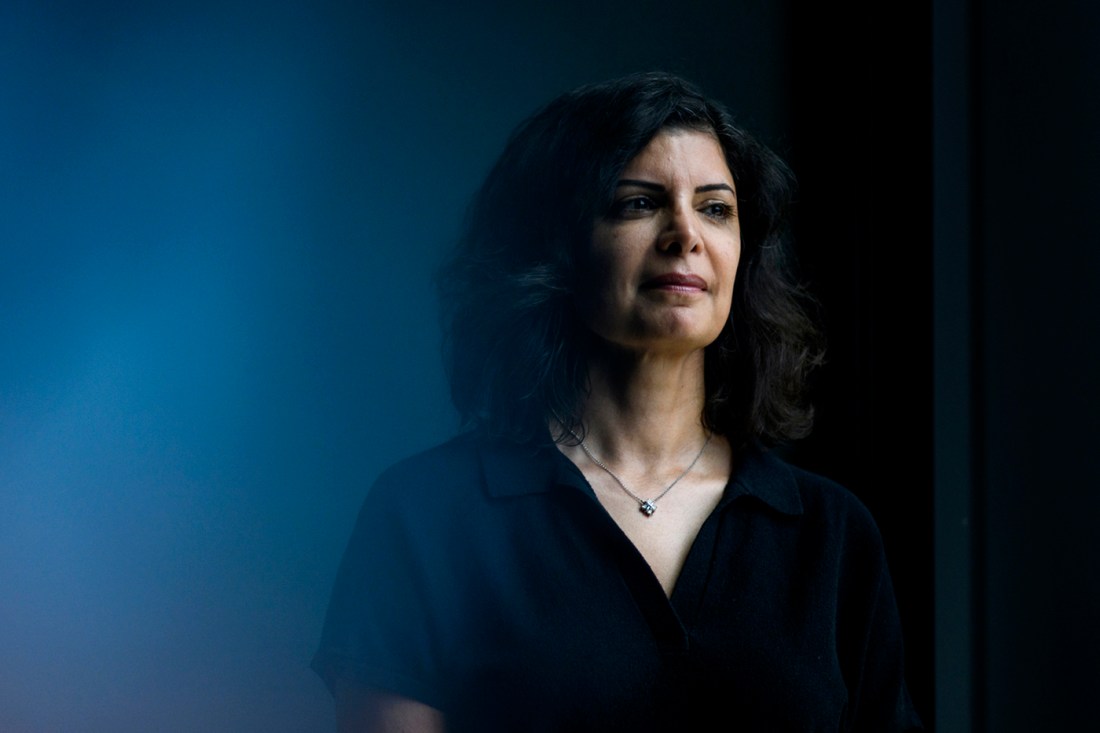
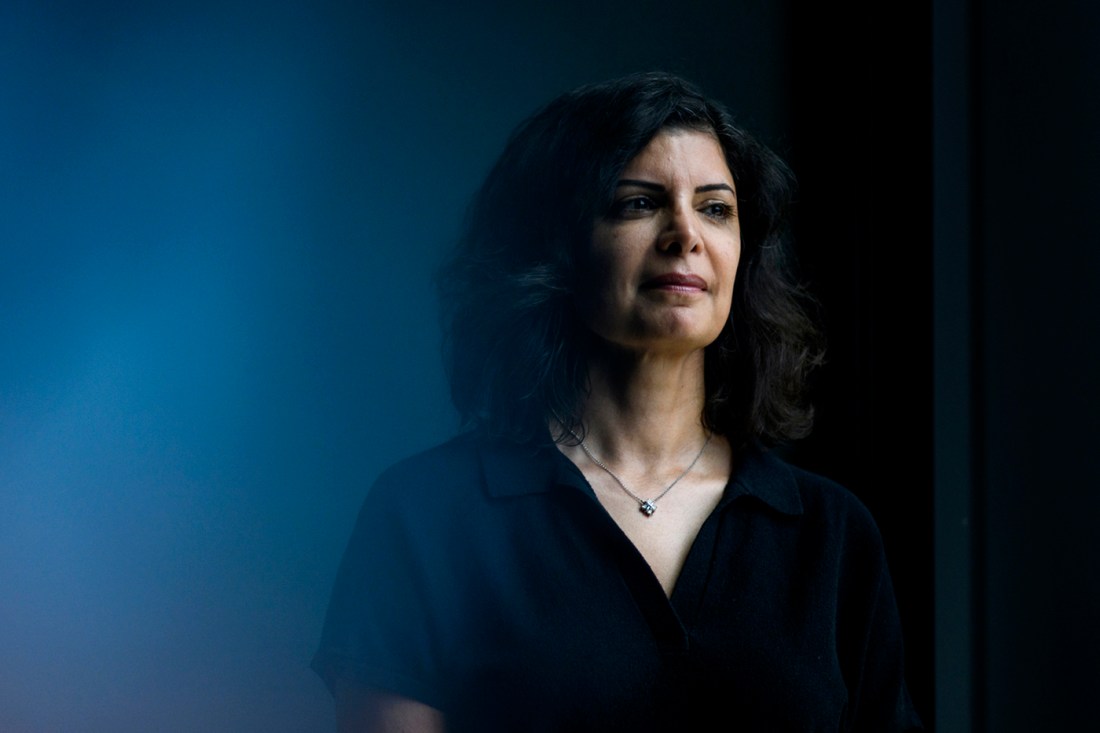
By involving social scientists in the process of building this tool, the team hopes to bring a human-centered approach to AI development that doesn't overlook humans amid the massive data set their tool is trained on.
“This model provides a much more complete reflection of the world as people experience it than many other models,” says Sune Lehmann, an author on the paper, who was recently published in Nature Computational Science.
At the heart of life2vec is the massive dataset that the researchers used to train their model. The data are held by Statistics Denmark, Denmark's central authority for statistics, and, although subject to strict regulation, can be accessed by some members of the public, including researchers. The reason it is so tightly controlled is that it includes a detailed register of every Danish citizen.
The many facts and figures that make up a life are spelled out in the data, from health factors and education to income. The researchers used this data to generate long-term patterns of repeated life events to feed their model, taking the transformer model approach used to train LLMs in language and adapting it for a human life represented as a sequence of events.
The whole history of a human life, in a way, can also be seen as a huge sentence of the many things that can happen to a human being,” says Lehmann, professor of network and complexity science at DTU Compute, Technical University. of Denmark and previously a postdoctoral fellow at Northeastern.
The model uses the information it learns from observing millions of sequences of life events to create what are called vector representations in embedding spaces, where it begins to categorize and make connections between life events such as factors of income, education or health. These embedding spaces serve as the basis for the predictions the model ends up making.
One of the life events the researchers predicted was a person's likelihood of mortality.
“When we visualize the space that the model uses to make predictions, it looks like a long roller that takes you from low probability of death to high probability of death,” says Lehmann. “Then we can show that at the end where there's a high probability of death, many of those people actually died, and at the end where there's a low probability of death, the causes of death are something we couldn't predict, like car accidents.”
The work also shows how the model is able to predict individual responses to a standard personality questionnaire, especially when it comes to extraversion.
Eliassi-Rad and Lehmann note that although the model makes very accurate predictions, these are based on correlations, highly specific cultural and social contexts, and the kinds of biases present in each data set.
“This kind of tool is like an observatory of society – and not of all societies,” says Eliassi-Rad. “This study was done in Denmark and Denmark has its own culture, its own laws and its own social norms. Whether that can be done in America is a different story.”
Given all these caveats, Eliassi-Rad and Lehmann see their predictive model less as a finished product and more as the beginning of a conversation. Lehmann says big tech companies have probably been building these kinds of predictive algorithms for years in locked rooms. He hopes this work can begin to create a more open, public understanding of how these tools work, what they're capable of, and how they should and shouldn't be used.
“The other way forward is to say, once we can make these accurate predictions about everything — because we've just picked two things, but we can predict all kinds of things — what are the ones that we want to apply to democratic societies?” says Lehmann. “I don't have those answers, but it's time to start the conversation because what we know is that detailed prediction of human lives is already happening and right now there's no discussion and it's happening behind closed doors.”
“It's about gaining knowledge instead of just making predictions,” adds Lehmann. “Knowledge is something we can share and something we can turn into action.”
One of the most promising areas where researchers see this tool having a positive impact is healthcare.
“I'm optimistic and I want to spend more time in this direction because I think we could really do good and we could really help by mining this space to help people,” says Lehmann. “This is not texting people saying, 'You're going to get cancer if you don't change,' but you can ask your doctor to get that information to help you. “
Eliassi-Rad says health care is also a promising application because it represents one of the areas of ethical concern that concerns her when it comes to how this technology is often implemented: accountability.
“I think health care is a good avenue to use this tool as an exploration and maybe to be able to provide better health care,” says Eliassi-Rad. “Specifically, it's appropriate because there are people who can be held accountable, as opposed to the absence of accountability when people's lives are ruined by some prediction that an artificial intelligence model makes.”
Eliassi-Rad wants to avoid the ethical pitfalls of how predictive tools have been used to influence policy in the past, such as in the case of a Dutch Fraud Assessment Algorithm. A tool like life2vec is less about predicting every aspect of a person's future and more about exploring trends in a society, its policies and its people at a level never before possible.
“It's not good to think of people as vectors in some Euclidean space and that's why it's more about exploring because if you start thinking of people as vectors (i.e. mathematical objects), mathematical objects come and go,” says Eliassi -Rad. “But they are real people –– they have hearts and minds.”